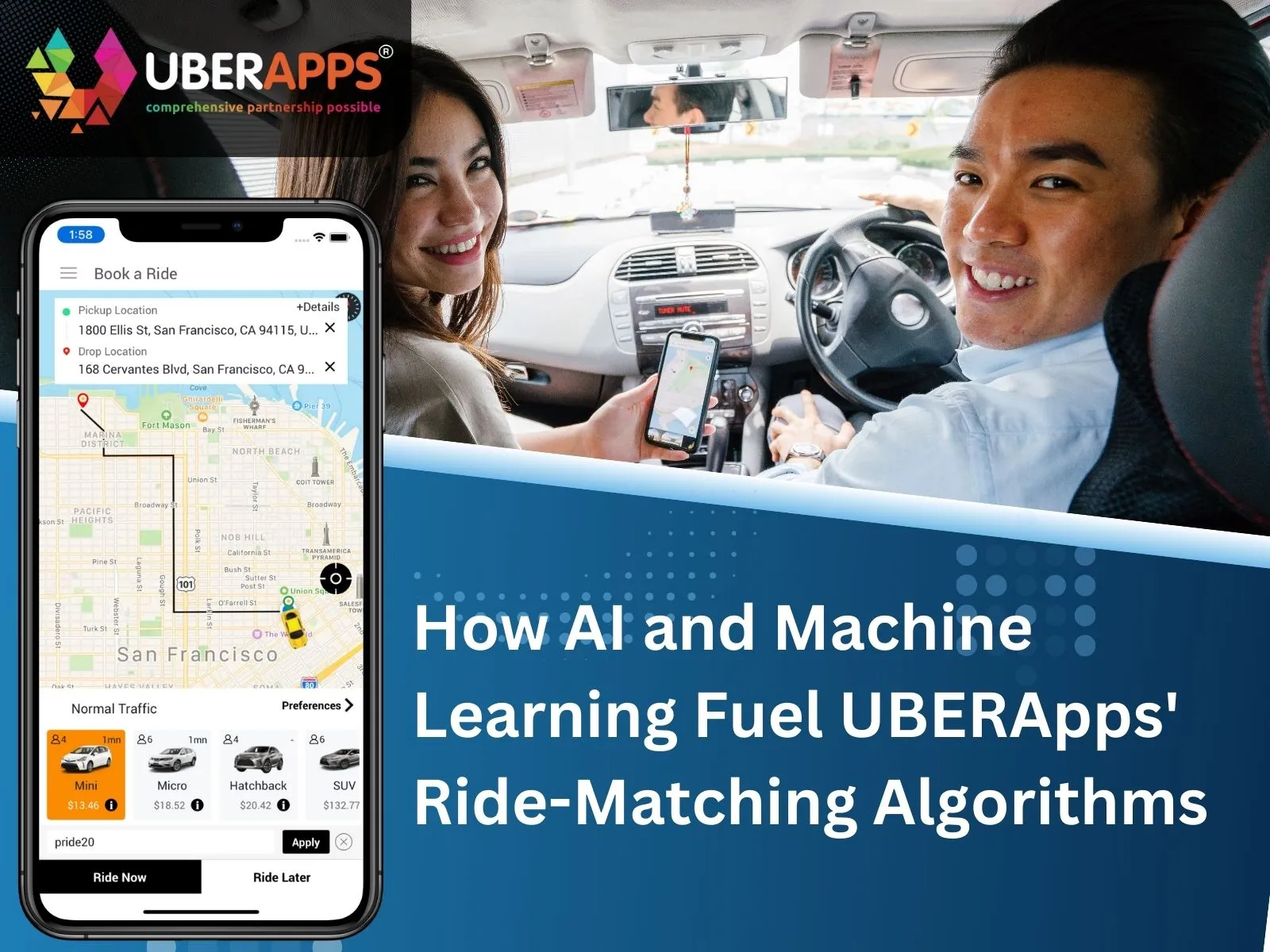
How AI and Machine Learning Fuel UBERApps' Ride-Matching Algorithms
In today's fast-paced world, convenience is king, and ride-sharing platforms like Uber have revolutionized how we get around. A significant part of this success can be credited to artificial intelligence (AI) and machine learning (ML). These technologies are the backbone of Uber's ride-matching algorithms, ensuring riders connect seamlessly with drivers in real time. But how exactly do AI and ML make this happen? In this article, we'll dive into the intricate workings of Uber's ride-matching algorithms and explore the critical role that AI and ML play in creating a smoother, faster, and more reliable ride-sharing experience.
UBERApps Technologies has emerged as a leader in taxi app development by integrating artificial intelligence (AI) and machine learning (ML) into its ride-matching algorithms. These technologies optimize demand forecasting, dynamic pricing, and route planning, ensuring seamless connections between riders and drivers. AI also enhances safety and fraud prevention, building user trust. With continuous innovation in AI and ML, UBERApps is setting new standards in the ride-sharing industry, positioning itself to shape the future of urban mobility with smarter, safer, and more reliable solutions.
1Understanding Ride-Matching Algorithms
At the heart of Uber's service is its ride-matching algorithm, a sophisticated system that pairs riders with nearby drivers efficiently. The algorithm's primary goal is to minimize the waiting time for riders while ensuring drivers get continuous rides without significant downtime. To achieve this, Uber relies heavily on AI and ML to predict demand, optimize routes, and manage dynamic pricing.
Ride-matching goes beyond simple proximity calculations. It involves analyzing multiple data points such as traffic conditions, driver availability, expected travel times, and rider preferences. AI models process this data in real time, making quick and accurate decisions to ensure a smooth experience for both riders and drivers.
2 The Role of Artificial Intelligence in Ride Matching
AI in Uber's ride-matching algorithms is about making smart predictions. By analyzing historical data and real-time inputs, AI helps forecast demand spikes, optimize driver positioning, and predict where the next surge in ride requests might occur.
For example, during peak hours or events, AI algorithms can predict high-demand areas and proactively direct drivers to those locations. This anticipation reduces wait times for riders and maximizes earnings for drivers. AI also helps in handling cancellations effectively, reallocating rides swiftly to minimize disruption.
3 Machine Learning: The Brain Behind Optimization
Machine learning is a subset of AI that allows Uber's systems to learn and improve over time. By analyzing vast amounts of historical ride data, ML models can identify patterns in user behavior, such as popular pick-up spots and the most efficient routes at different times of the day.
Uber's ML algorithms continuously refine their predictions based on feedback and new data. For instance, if a particular route consistently experiences traffic congestion, the system learns to adjust its route suggestions dynamically. This self-learning ability is crucial for providing accurate ETAs (estimated times of arrival) and improving the overall efficiency of ride-matching.
4Predictive Analytics in Demand Forecasting
Predictive analytics is an essential tool powered by AI and ML in Uber's ecosystem. By analyzing historical data, including previous ride requests, weather conditions, local events, and time-of-day trends, Uber can predict when and where demand will surge.
This foresight allows the platform to ensure enough drivers are available in high-demand areas, reducing wait times and cancellations. For instance, if the system predicts a spike in demand near a stadium post-game, it will alert drivers nearby to position themselves advantageously. Predictive analytics thus helps balance supply and demand efficiently.
5 Dynamic Pricing: Surge as a Data-Driven Solution
One of Uber's most controversial yet effective features is surge pricing, heavily reliant on AI and ML. Surge pricing adjusts fares based on real-time demand and supply. When the demand for rides in a particular area outstrips the available drivers, prices automatically increase.
The AI algorithms behind this system assess multiple factors such as the number of requests, available drivers, and traffic conditions to set a fair price that incentivizes more drivers to move to the surge area. While surge pricing can sometimes lead to higher fares, it plays a critical role in balancing the ride ecosystem, ensuring availability when it's needed most.
6 Route Optimization Using AI and ML
Efficient route planning is crucial for reducing travel time and fuel costs, directly impacting rider satisfaction and driver profitability. Uber's algorithms use AI and ML to analyze traffic data, road closures, and historical patterns to suggest optimal routes.
By leveraging these technologies, Uber can adjust routes in real time, helping drivers avoid traffic jams and construction zones. Additionally, AI-based route optimization considers factors such as left turns (which often cause delays) and high-risk intersections to enhance safety and efficiency.
7NLP and AI-Powered Communication
Natural Language Processing (NLP), a branch of AI, plays a significant role in enhancing user experience on the Uber app. Through NLP, Uber's chatbots handle common inquiries and issues swiftly, providing real-time support without human intervention.
For instance, when a rider reports an issue like a lost item, AI-driven systems use NLP to understand the query, initiate a resolution process, and keep the user informed. This automated support reduces response times and improves overall customer satisfaction.
8Fraud Detection and Prevention with AI
With a massive number of transactions occurring every day, fraud detection is critical for Uber. AI models analyze patterns in transaction data to detect anomalies such as unusual payment methods or trip routes that may indicate fraudulent activity.
Machine learning algorithms are particularly effective here as they learn from previous fraud cases to improve detection accuracy continuously. This proactive approach minimizes losses and maintains trust in the platform.
9Enhancing Driver and Rider Safety
Safety is a top priority for Uber, and AI contributes significantly to this aspect. AI algorithms monitor driving patterns and flag behaviors like hard braking, rapid acceleration, or excessive speeding. Drivers receive feedback on their driving habits, encouraging safer practices.
Additionally, Uber uses AI to verify driver identities through real-time selfie verification, ensuring the driver matches the registered profile. This feature prevents unauthorized use of driver accounts and enhances rider security.
10 The Future of AI and ML in Uber's Ride-Matching
Looking ahead, the integration of AI and ML in Uber's ride-matching algorithms will only deepen. With advancements in deep learning and real-time data analytics, we can expect even more accurate demand forecasting, route optimization, and dynamic pricing.
Moreover, the potential for autonomous vehicles, powered by AI, represents a significant leap forward. As Uber continues to invest in AI research, the goal of achieving a fully autonomous ride-sharing network seems increasingly attainable.
Conclusion
In a world where convenience drives customer preferences, UBERApps Technologies has positioned itself as a leader in taxi app development by leveraging the power of artificial intelligence (AI) and machine learning (ML). By adopting these advanced technologies, UBERApps has successfully transformed the ride-sharing experience, offering seamless, efficient, and reliable solutions that benefit riders and drivers.
From sophisticated ride-matching algorithms that minimize wait times to predictive analytics that forecast demand surges, UBERApps' platforms demonstrate how AI and ML can elevate the standard of ride-sharing services. The integration of dynamic pricing and real-time route optimization further showcases UBERApps' commitment to harnessing AI to enhance user satisfaction and profitability for drivers.
Additionally, UBERApps' focus on safety and fraud prevention through AI-driven monitoring and verification systems reflects its dedication to building trust and reliability in the ride-sharing ecosystem. As AI and ML technologies evolve, UBERApps is well-positioned to lead the way with cutting-edge solutions that redefine the future of ride-sharing.
By continually refining its algorithms and expanding its AI capabilities, UBERApps is not just keeping pace with industry giants like Uber but is setting new standards in the taxi app development space. As we move forward, the company's ability to innovate and adapt will undoubtedly play a crucial role in shaping the future of urban mobility.
With a strong foundation in AI and ML, UBERApps Technologies is ready to drive the next wave of innovation in ride-sharing, making every ride smarter, safer, and more convenient
FAQS
1. How does AI predict demand for Uber rides?
AI predicts demand by analyzing historical data, current trends, local events, and weather conditions to forecast where and when ride requests are likely to surge
2. What is surge pricing and how does AI manage it?
Surge pricing is an AI-driven feature that adjusts fares based on real-time demand and supply, encouraging more drivers to service high-demand areas.
3. How does machine learning improve route optimization for Uber?
Machine learning analyzes traffic patterns and previous route data to suggest the most efficient paths, minimizing delays and fuel costs.
4. What role does NLP play in Uber's service?
NLP powers Uber's chatbots, enabling them to handle user inquiries efficiently and improve customer service experiences.
5. How does AI enhance safety for Uber riders and drivers?
AI monitors driving behaviors, verifies driver identities, and analyzes route risks to ensure a safer experience for all users.
Author's Bio
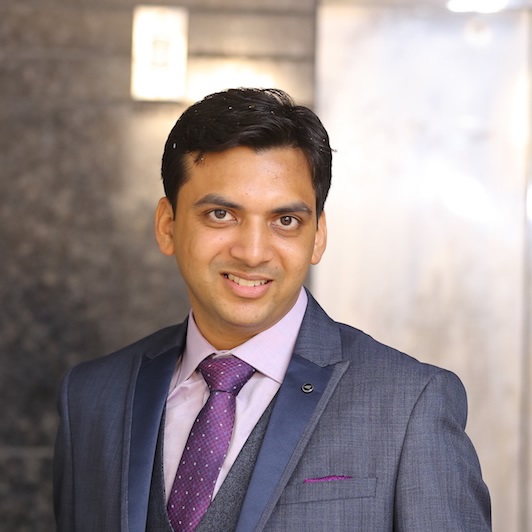
Vinay Jain is the Founder of UBERApps and brings over 10 years of entrepreneurial experience. His focus revolves around software & business development and customer satisfaction.